Tutorials at ISCAS 2022
This year a major emphasis has been placed on providing a comprehensive tutorial program for participants of the conference. The tutorial topics are focused primarily on emerging technologies that should be of widespread interests to the CAS Community. Included are eight tutorials on basic and advanced machine learning, a track of four tutorials on vehicular electronics and radiation hard circuits, a track of four tutorials on data converters, and a track of four tutorials on secure integrated circuits and power management.
A total of 20 tutorials will be presented by experts in the field. The tutorials will be given on Sunday May 29th. Each tutorial is scheduled in a 90- minute time slot with two tutorials sessions in the morning and two tutorial sessions in the afternoon. As such, in-person registrants can attend up to four tutorials on Sunday. Recordings will be made of the tutorials so all registrants to the tutorial program will be able to view streams of any of the tutorials for 30 days after the conference is over. A single tutorial registration fee provides access to all tutorials.
The 20 tutorials will be arranged into 5 tracks but participants can move from one track to another throughout the day. A poll will be sent to tutorial registrants in early May to obtain an estimate of how many participants can be expected in each tutorial to help facilitate determining the size of the rooms that will be needed for each tutorial.
Tutorial co-chairs:
Gabriele Manganaro, Mediatek
John Khoury, Silicon Labs
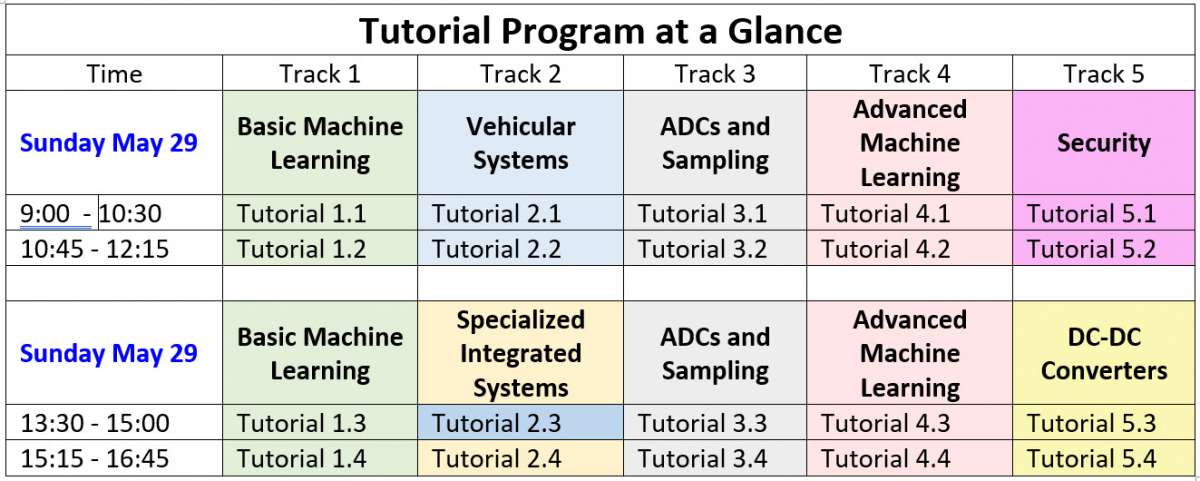


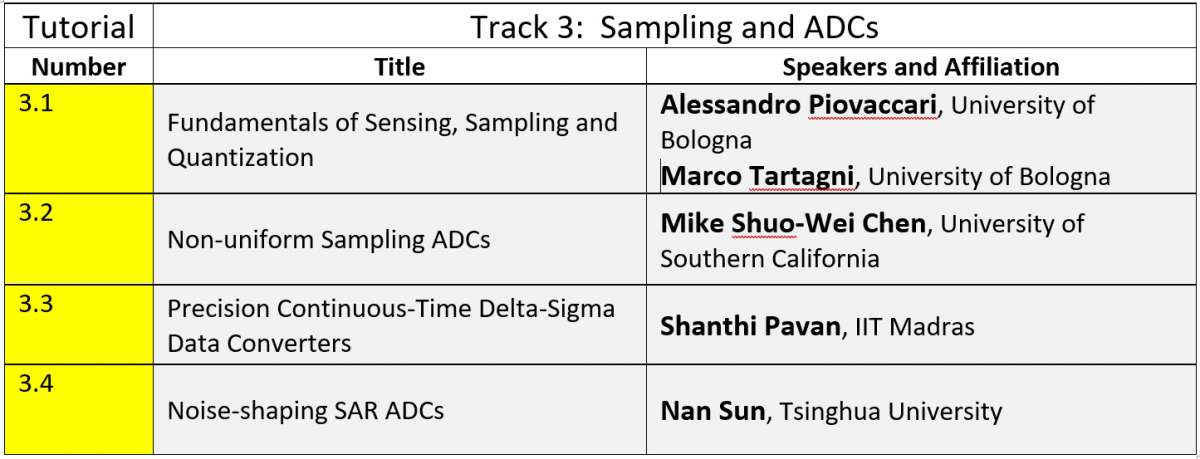

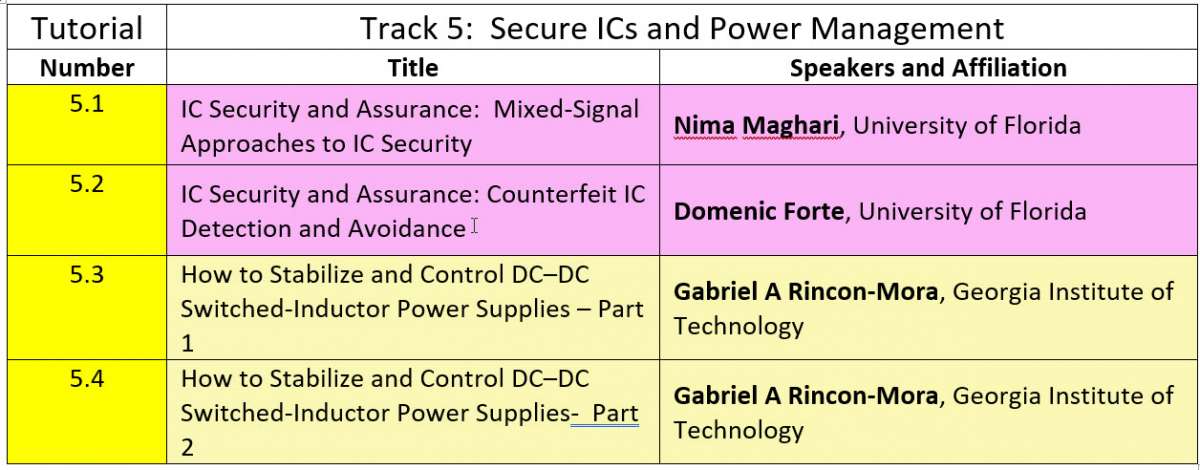
Meet The Tutorial Speakers
Track 1 Speakers
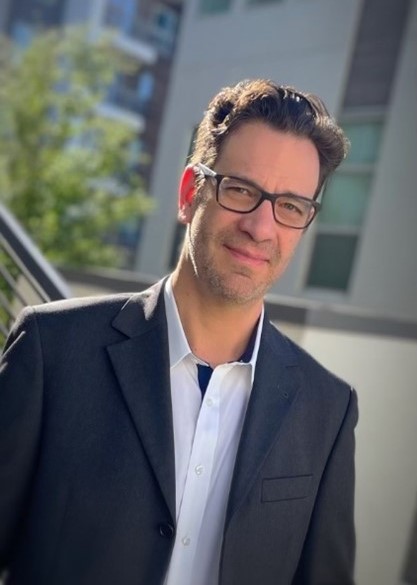
Javier Elenes
Silicon Laboratories
Tutorial 1.1 Introduction to Machine Learning
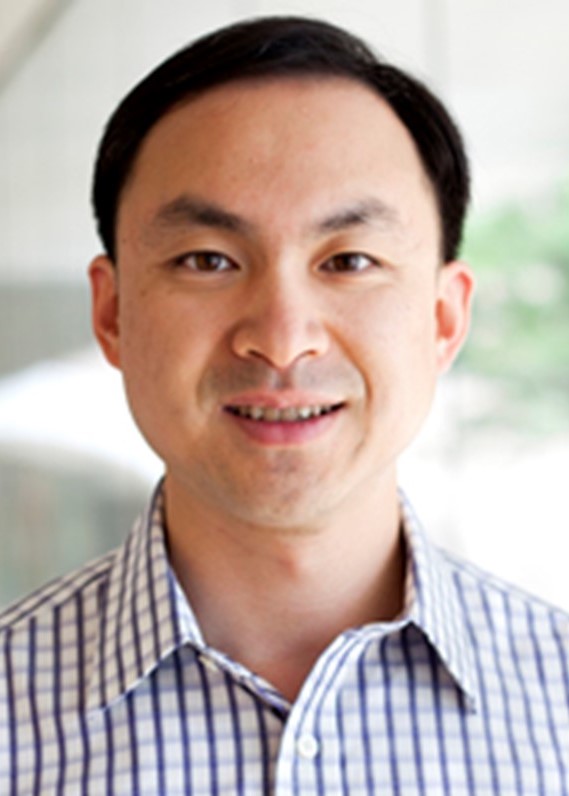
Zhengya Zhang
Universithy of Michigan
Tutorial 1.2 Machine Learning Hardware Design for Efficieny, Flexibility, and Scalability
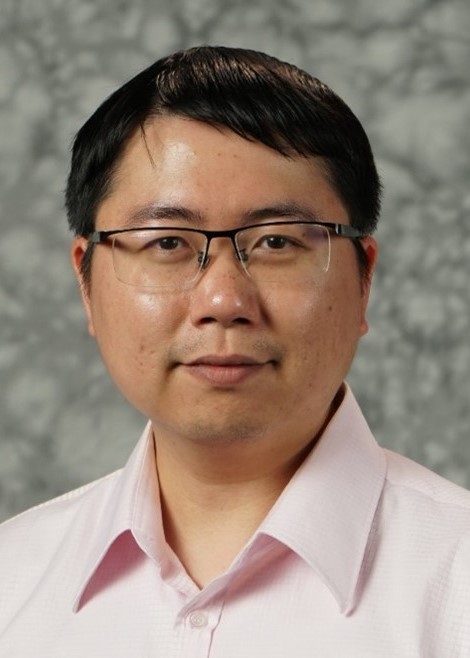
Shimeng Yu
Georgia Institute of Technilogy
Tutorial 1.3 Compute-in-Memory
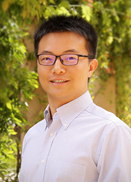
Song Han
Massachusetts Institute of Technology
Tutorial 1.4 Tiny Machine Learning
Track 2 Speakers
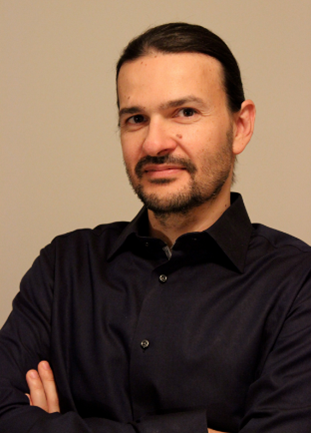
Kostas Doris
NXP Semiconductors
Tutorial 2.1 Mm-wave Radar and Power Electronics for Automotive Applications
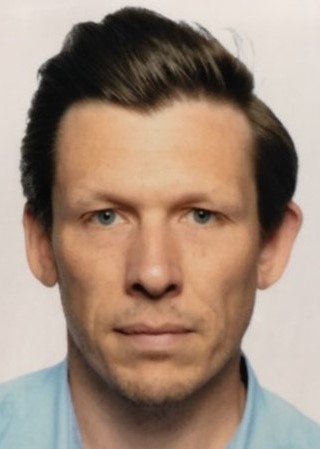
Ilja Ocket
IMEC Belgium
Tutorial 2.2 Mm-Wave Radars Beyond 100 GHz, Neuromorphic Artificial Intelligence and Links to 6G
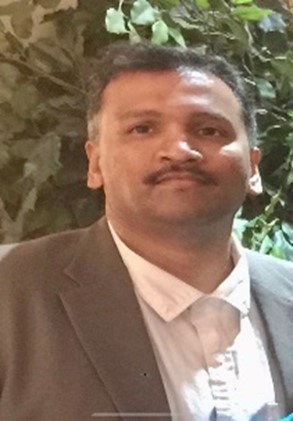
Sri Navaneeth Easwaran
Texas Instruments and Univ. of Erlangen-Nuremberg
Tutorial 2.3 Large Scale Integration of Analog, Mixed Signal and Power Integrated Circuits for Automotive Applications and Testing Methodology for Quality
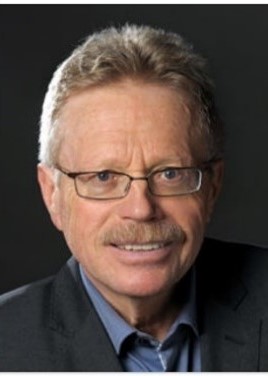
Robert Weigel
Univ. of Erlangen-Nuremberg
Tutorial 2.3 Large Scale Integration of Analog, Mixed Signal and Power Integrated Circuits for Automotive Applications and Testing Methodology for Quality
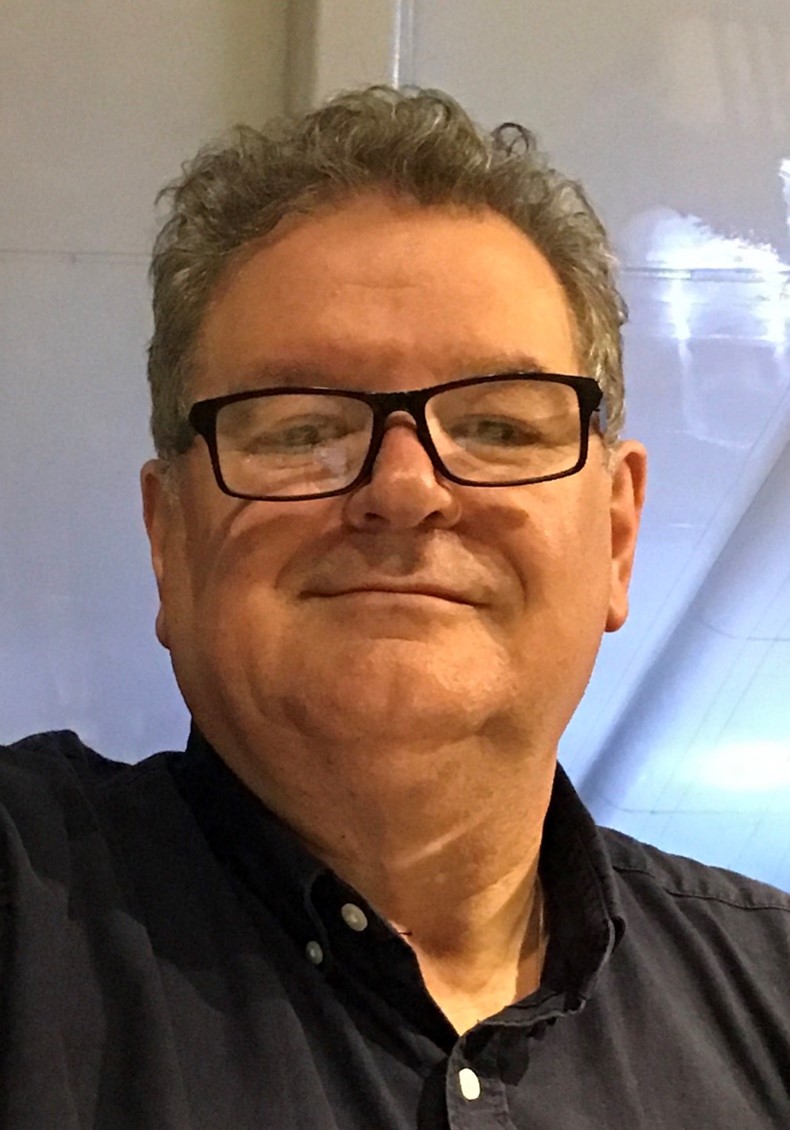
Ricardo Reis
Universidade Federal do Rio Grande do Sul, Brazil
Tutorial 2.4 Assessment and Mitigation of Radiation Effects on Circuits and Systems at Different Levels of Abstraction
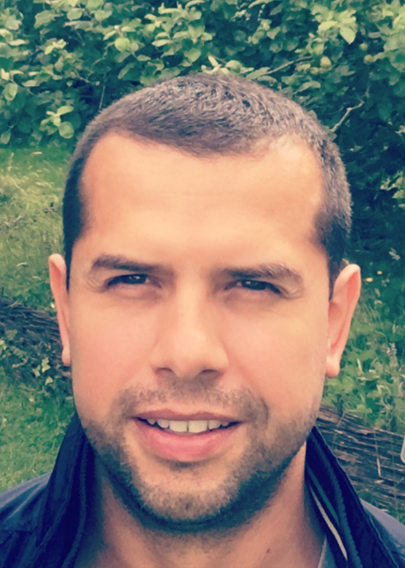
Luciano Ost
Wolfson School, Loughborough University, United Kingdom
Tutorial 2.4 Assessment and Mitigation of Radiation Effects on Circuits and Systems at Different Levels of Abstraction
Track 3 Speakers
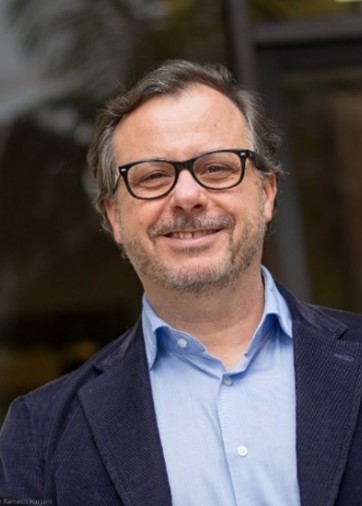
Alessandro Piovaccari
Univ. of Bologna
Tutorial 3.1 Fundamentals of Sensing, Sampling, and Quantization
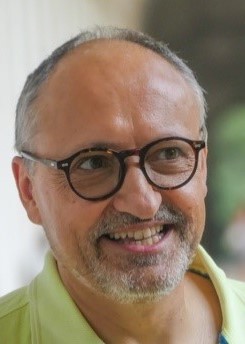
Marco Tartagni
Univ. of Bologna
Tutorial 3.1 Fundamentals of Sensing, Sampling, and Quantization
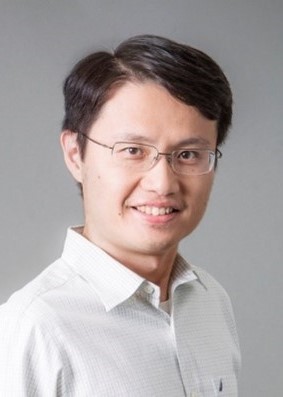
Mike Shuo-Wei Chen
Univ. of Southern California
Tutorial 3.2 Non-uniform Sampling ADCs
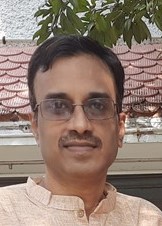
Shanthi Pavan
IIT Madras
Tutorial 3.3 Precision Continuous-Time Delta-Sigma Data Converters
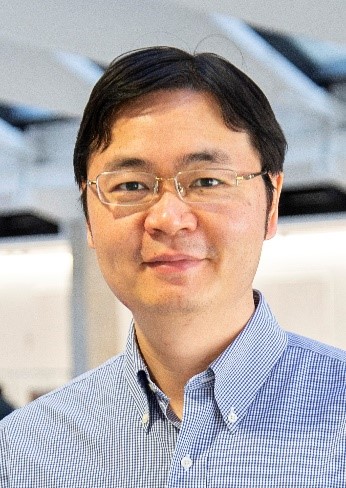
Nan Sun
Tsinghua University
Tutorial 3.4 Noise-Shaping SAR ADCs
Track 4 Speakers
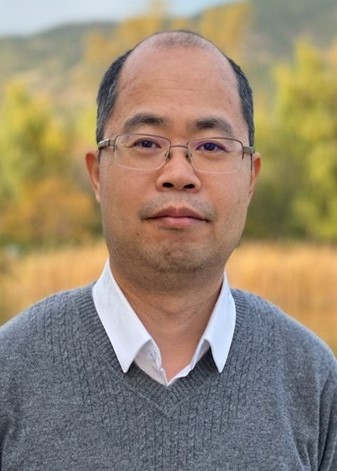
Zhibo Chen
Univ. of Science and Technology, China
Tutorial 4.1 Advances in Generative Image Compression
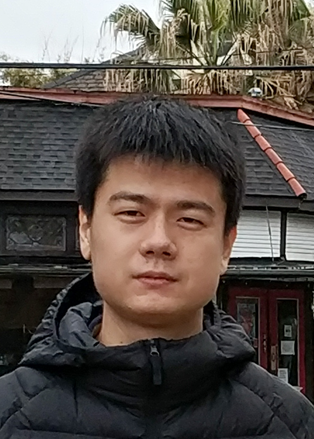
Jianxin Lin
Hunan University, China
Tutorial 4.2 Advances in Cross-Domain Image Generation
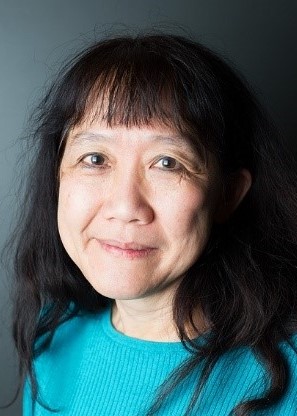
Shih-Chii Liu
University of Zurich and ETH Zurich
Tutorial 4.3 Neuromorphic Spiking Sensors, Network Accelerators, Non-volatile Memory Devices for Low-Power Edge Applications - Part I
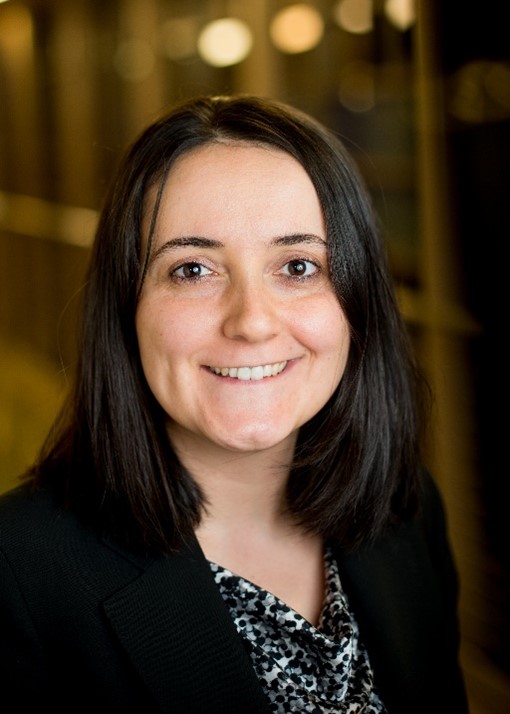
Duygu Kuzum
Univ. of California, San Diego
Tutorial 4.3 Neuromorphic Spiking Sensors, Network Accelerators, Non-volatile Memory Devices for Low-Power Edge Applications - Part I
Tutorial 4.4 Neuromorphic Spiking Sensors, Network Accelerators, Non-volatile Memory Devices for Low-Power Edge Applications - Part II
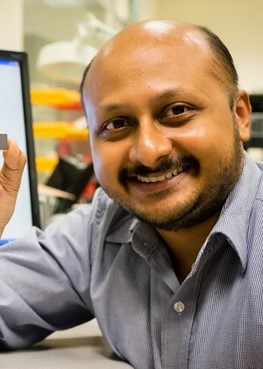
Arindam Basu
City University of Hong Kong
Tutorial 4.4 Neuromorphic Spiking Sensors, Network Accelerators, Non-volatile Memory Devices for Low-Power Edge Applications - Part II
Track 5 Speakers
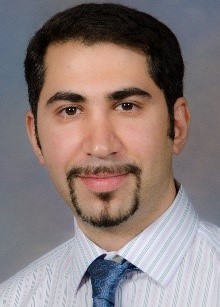
Nima Maghari
Univ. of Florida
Tutorial 5.1 IC Security and Assurance: Mixed-Signal Approaches to IC Security
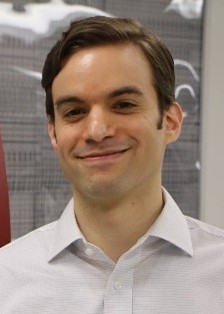
Domenic Forte
Univ. of Florida
Tutorial 5.2 IC Sercuirty and Assurance: Counterfeit IC Detection and Avoidance
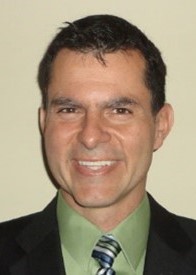
Gabriel A Rincon-Mora
Georgia Institute of Technology
Tutorial 5.3 How to Stabilize and Control DC-DC Switched-Inductor Power Supplies - Part 1
Tutorial 5.4 How to Stabilize and Control DC-DC Switched-Inductor Power Supplies - Part 2
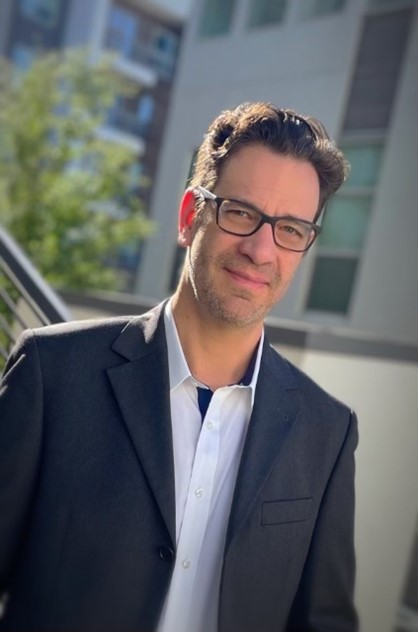
Javier Elenes
Tutorial 1.1 Introduction to Machine Learning
Abstract
Learning is the acquisition of knowledge or skills through experience, study, or being thought. Machine learning is the automated discovery of rules to execute a data processing task given examples of what is expected. Deep Learning is a sub-field of Machine Learning in which transformations are applied to input data using multiple processing layers. This tutorial is organized as follows. Part 1 introduces Machine Learning and Deep Learning, Deep learning models, model training, and how to build and test models using readily available open-source tools. Part 2 introduces Convolutional Neural Networks, Unsupervised Learning, and Autoencoders. Various examples of applications and use cases are provided throughout the tutorial.
Bio
Javier Elenes received Bachelor of Science and Master of Science degrees in electrical engineering from Drexel University in 1996. He joined Silicon Labs in 2004 where he currently serves as Distinguished Engineer. From 2004 until 2016 he led the development and implementation of various digital signal processing algorithms for receiver synchronization, signal detection, channel estimation, adaptive equalization, and antenna diversity. From 2016 until 2019 he focused on security, side-channel analysis, device hacking and countermeasures. Since 2019 he has been working on Deep Learning models and architectures for embedded devices. He holds 33 U.S. patents in signal processing, receiver architectures, and embedded security.
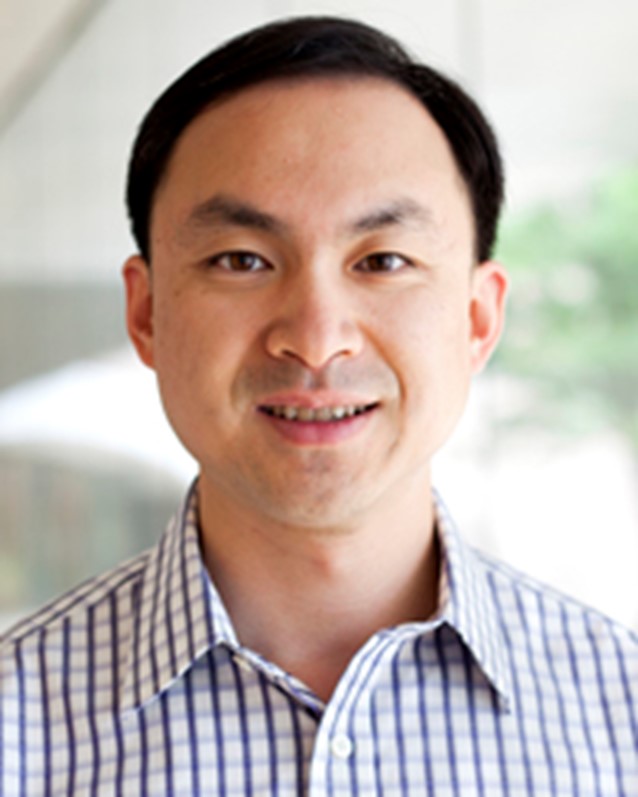
Zhengya Zhang
Tutorial 1.2 Machine Learning Hardware Design for Efficiency, Flexibility and Scalability
Abstract
Machine learning (ML) is the driving application of the next-generation computational hardware. How to design ML hardware to achieve a high performance, efficiency, and flexibility to support fast growing ML workloads is a key challenge. Besides dataflow-optimized systolic arrays and single instruction, multiple data (SIMD) engines, efficient ML accelerators have been designed to take advantage of static and dynamic data sparsity. To accommodate the fast-evolving ML workloads, matrix engines can be integrated with an FPGA to provide the efficiency of kernel computation and the flexibility of control. To support the increasing ML model complexity, modular chiplets can be tiled on a 2.5D interposer and stacked in a 3D package. We envision that a combination of these techniques will be required to address the needs of future ML applications.
Bio
Zhengya Zhang received the B.A.Sc. degree from the University of Waterloo in Canada in 2003, and the M.S. and Ph.D. degrees from UC Berkeley in 2005 and 2009. He has been a faculty member at the University of Michigan, Ann Arbor since 2009. His research is in low-power and high-performance integrated circuits and systems for computing, communications, and signal processing. Dr. Zhang was a recipient of the NSF CAREER Award, the Intel Early Career Faculty Award, the Neil Van Eenam Memorial Award from the University of Michigan, and the David J. Sakrison Memorial Prize from UC Berkeley. He serves on the program committees of the Symposia on VLSI Technology and Circuits and CICC, and the editorial board of the IEEE Transactions on VLSI Systems.
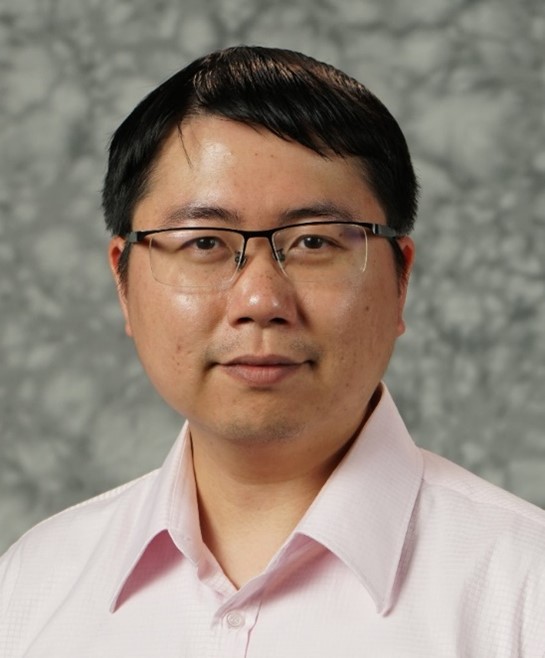
Shimeng Yu
Tutorial 1.3 Compute-in-Memory
Abstract
Compute-in-memory (CIM) is a new paradigm for machine learning hardware acceleration. DNN+NeuroSim is an integrated framework to benchmark CIM accelerators for deep neural network (DNN), with hierarchical design options from device-level, to circuit-level and up to algorithm-level. NeuroSim is a C++ based circuit-level macro model, which can achieve fast early-stage pre-RTL design exploration (compared to a full SPICE simulation). It takes design parameters including memory types (includes SRAM, RRAM, PCM, MRAM and FeFET), non-ideal device parameters, transistor technology nodes (from 130 nm to 7nm), memory array size, training dataset and traces to estimate the area, latency, dynamic energy, leakage power. A python wrapper is developed to interface NeuroSim with deep learning platforms Pytorch, to support flexible network topologies including VGG, DenseNet and ResNet for CIFAR/ImageNet classification. It supports weight/activation/gradient/error quantization in algorithm, and takes non-ideal properties of synaptic devices and peripheral circuits, in order to estimate training/inference accuracy. The framework is open-sourced and publicly available on GitHub https://github.com/neurosim/. NeuroSim’s user community is growing. This tutorial aims for a broader education to the community, and help the researchers to use/modify the code more flexibly for their own research purposes.
Bio
Shimeng Yu is currently an associate professor of electrical and computer engineering at Georgia Institute of Technology. He received the B.S. degree in microelectronics from Peking University in 2009, and the M.S. degree and Ph.D. degree in electrical engineering from Stanford University in 2011 and 2013, respectively. From 2013 to 2018, he was an assistant professor at Arizona State University. Prof. Yu’s research interests are the semiconductor devices and integrated circuits for energy-efficient computing systems. His research expertise is on the emerging non-volatile memories for applications such as deep learning accelerator, in-memory computing, 3D integration, and hardware security. Among Prof. Yu’s honors, he was a recipient of NSF Faculty Early CAREER Award in 2016, IEEE Electron Devices Society (EDS) Early Career Award in 2017, ACM Special Interests Group on Design Automation (SIGDA) Outstanding New Faculty Award in 2018, Semiconductor Research Corporation (SRC) Young Faculty Award in 2019, ACM/IEEE Design Automation Conference (DAC) Under-40 Innovators Award in 2020, IEEE Circuits and Systems Society (CASS) Distinguished Lecturer for 2021-2022, and IEEE EDS Distinguished Lecturer for 2022-2023, etc. He is a senior member of the IEEE.
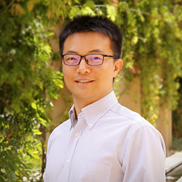
Song Han
Tutorial 1.4 Tiny Machine Learning
Abstract
Modern deep learning requires a massive amount of computational resources, carbon footprint, and engineering efforts. On mobile devices, the hardware resource and power budget are very limited, and on-device machine learning is challenging; retraining the model on-device is even more difficult. We make machine learning efficient and fit tiny devices (TinyML). Our research is highlighted by full-stack optimizations, including the neural network topology, inference library, and the hardware architecture, which allows a larger design space to unearth the underlying principles.
Bio
Song Han is an assistant professor in MIT's Department of Electrical Engineering and Computer Science. His research focuses on efficient deep learning computing. He has proposed “deep compression” as a way to reduce neural network size by an order of magnitude, and the hardware implementation “efficient inference engine” that first exploited model compression and weight sparsity in deep learning accelerators. He has received best paper awards at the International Conference on Learning Representations and Field-Programmable Gate Arrays symposium. He is also a recipient of an NSF Career Award and MIT Tech Review's 35 Innovators Under 35 award. Many of his pruning, compression, and acceleration techniques have been integrated into commercial artificial intelligence chips. His team’s work on hardware-aware neural architecture search that bring deep learning to IoT devices was highlighted by MIT News, Wired, Qualcomm News, VentureBeat, IEEE Spectrum, integrated in PyTorch and AutoGluon, and received many low-power computer vision contest awards. He earned a PhD in electrical engineering from Stanford University.
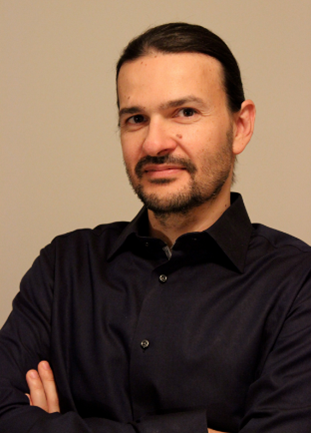
Kostas Doras
Tutorial 2.1 Mm-wave CMOS Automotive Radar: from basic theory to high performance implementations
Abstract
Mm-wave automotive radar is essential sensor technology for full drive automation and evolves steadily in the 76-81GHz band. Mm-wave CMOS in particular is becoming nowadays the mainstream technology platform for high performance car radar sensors in all application segments. The combination of highly integrated RFCMOS transceivers having ever increasing levels of performance with automotive grade nano-meter scale microprocessors enables radar resolution boost in range, velocity and angle, enhanced sensing robustness and large and cost-effective sensor diversity from high performance imaging radars to corner radars.
This tutorial module covers mm-wave automotive radar from basic principles of ranging and detection of angle of arrival to waveform choices for MIMO, circuit requirements and RFCMOS architectures. Examples of high performance automotive transceivers will be shown and the emerging landscape to frequencies beyond 100GHz will be sketched.
Bio
Kostas Doris is Fellow at NXP Semiconductors and part time Professor at Technical University of Eindhoven. Kostas is the (co-) author of several papers, patents, and books in the field of data converters. His is currently leading RFCMOS automotive radar transceiver innovation activities in NXP Semiconductors. Kostas Doris served IEEE as Associate Editor for the IEEE Transactions of Circuits and Systems, and as Technical Program Committee and Regional EU Chair for the International Solid State Circuits Conference.
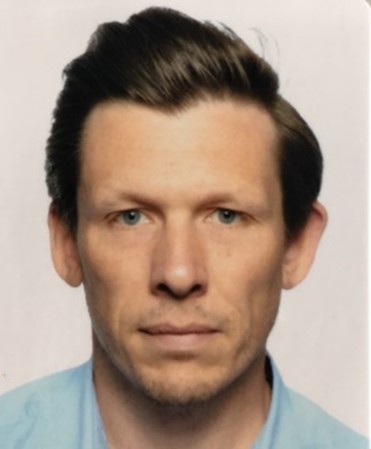
Ilja Ocket
Tutorial 2.2 Mm-Wave Radars Beyond 100 GHz, Neuromorphic Artificial Intelligence and Links to 6G
Abstract
Automotive radar sensors have been at the core of advanced driver assistance systems and represent a reliable perception baseline towards autonomous driving. Giving cars full 360 degree sensing at medium/long range is today possible at acceptable cost due to advances in millimeter-wave CMOS transceivers. Angular resolution is ultimately limited by the number of transmit and receive antennas that can be physically integrated in a given aperture, but also by the amount of data processing that needs to be performed. For these reasons, imec is developing 140 GHz CMOS radar front-ends (smaller antennas), but also on neuromorphic compute architectures and algorithms to address these challenges.
This tutorial covers the technologies that are under development for 140 GHz CMOS radar, which range from analog front-end circuitry to MMIC packaging, interconnect and antennas. We will also cover emerging neuromorphic AI technologies and how they relate to radar. Finally, we will touch on technology developments for 6G above 100 GHz, as these are expected to cross over to radar.
Bio
Ilja Ocket is a program manager at imec (Leuven, Belgium). He has a technical background in millimeter wave antenna and system-in-package technologies. He leads the radar and neuromorphic sensing programs at imec, with a focus on multi-sensor perception and Artificial Intelligence for robotics.
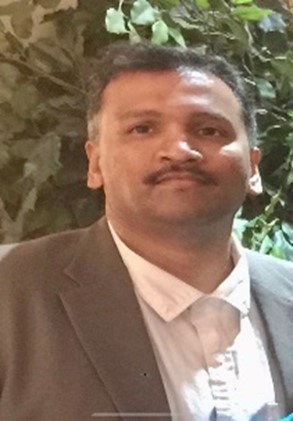
Sri Navaneeth Easwaran
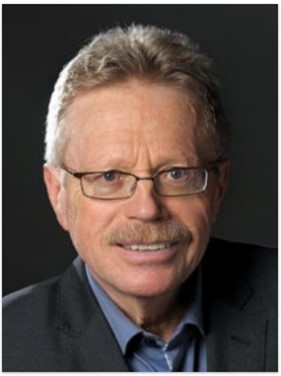
Robert Weigel
Tutorial 2.3 Large Scale Integration of Analog, Mixed Signal and Power Integrated Circuits for Automotive Applications and Testing Methodology for Quality
Abstract
Power transistors form the main component of automotive Integrated Circuits (ICs) and they handle several amperes of current (>2A). State of the Art is to integrate several power MOSFETs along with their gate drivers (including charge pumps or boost converters that supply the gate drivers) whose operating voltage range is from 5V to 60V. Reliability of these integrated gate drivers and power transistors is a key factor to meet the high-quality demands of the automotive applications. In this tutorial, challenges related to the design and reliability of circuits like LDOs, High Side (HS) drivers, Low Side (LS) drivers and configurable HS/ LS drivers are discussed along with information related to floating nodes, aging and reliability related concerns like NBTI/PBTI, HCI etc. and proven design techniques to simulate and mitigate these challenges. These gate drivers have to be thoroughly designed for robustness w.r.to. Electrical and Thermal Safe Operating Area (SOA) and its test methodology by shorting the outputs to ground and battery will be discussed. In this tutorial, Design FMEA (Failure Mode Effect Analysis) based analysis to mitigate risks at design and system level along with test concepts towards very low dppm (defective parts per million) will be discussed.
Bios
IDr. –Ing. Sri Navaneeth Easwaran, Senior Member IEEE, received his Bachelor’s (1998, Bharathidasan University), Master’s (2006, University Twente) degrees in Electrical Engineering and Dr. –Ing. degree from University of Erlangen-Nuremberg in 2017. He worked at SPIC Electronics, STMicroelectronics, Philips Semiconductors between 1998 and 2006. From 2006 he is with Texas Instruments (TI) where he was the design lead of airbag squib driver ICs and System Basis Chips. He is an IET Fellow (Feb 2021), TI Senior Member Technical Staff, has 20+ granted patents and 14 publications. He has offered tutorials on automotive ICs at IEEE Conferences. Since Dec 2020, he is offering iDLP (Industrial Distinguished Lecturer Program) CASS seminars on smart automotive circuits.
Dr. -Ing. Dr.-Ing. habil. Robert Weigel, Fellow IEEE and Fellow ITG, is Full Professor at the University of Erlangen-Nuremberg, Germany. He co-founded several companies some of which were later overtaken by Infineon, Intel and Apple, respectively. He has been engaged in microwave electronic circuits and systems and has published more than 1200 papers. He received the 2002 VDE ITG-Award, the 2007 IEEE Microwave Applications Award, the 2016 IEEE MTT-S Distinguished Educator Award, the 2018 Distinguished Service Award of the EuMA and the 2018 IEEE Rudolf Henning Distinguished Mentoring Award. He has been Distinguished Microwave Lecturer, MTT-S AdCom Member, and the 2014 MTT-S President.
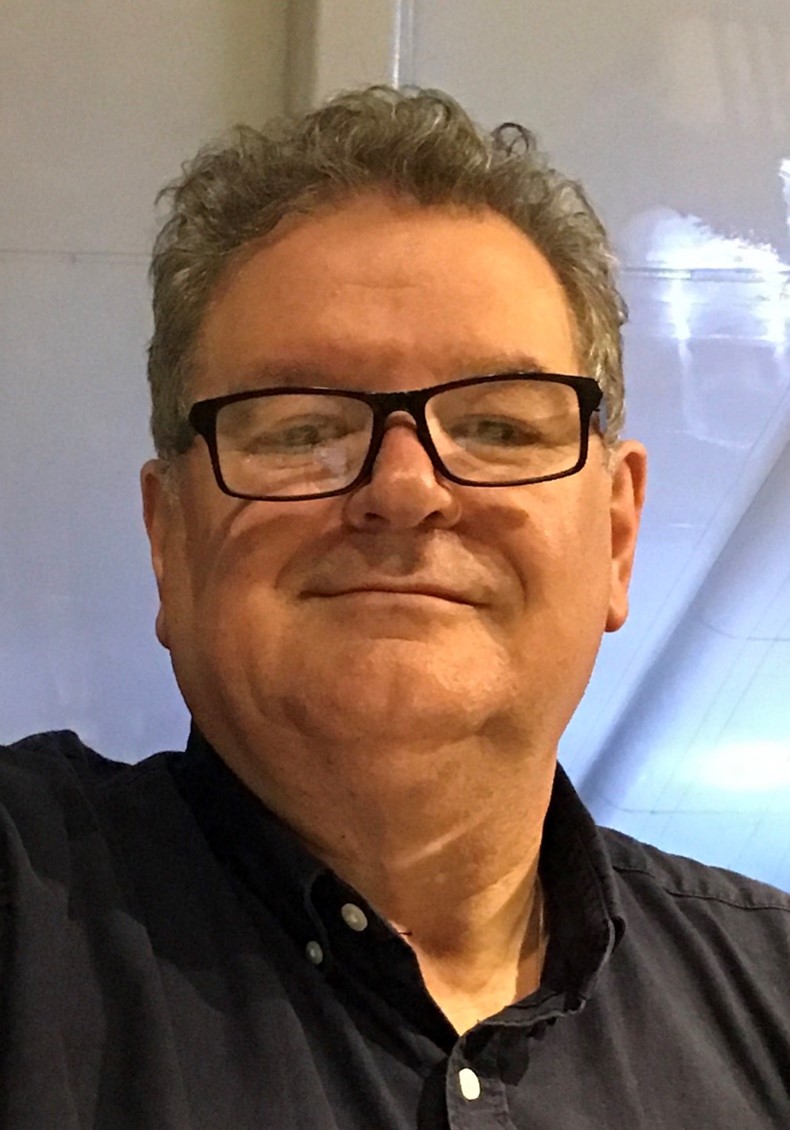
Ricardo Reis
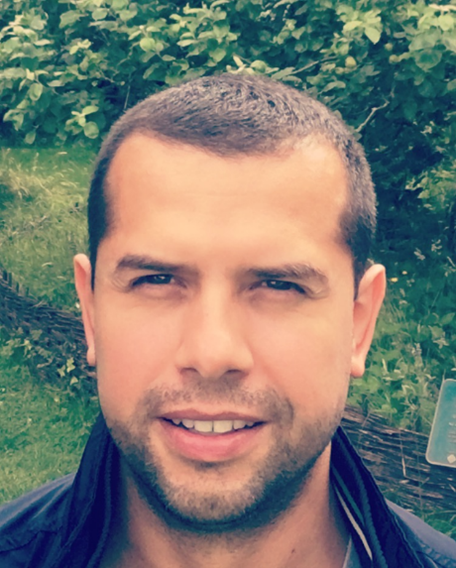
Luciano Ost
Tutorial 2.4 Assessment and Mitigation of Radiation Effects on Circuits and Systems at Different Levels of Abstraction
Abstract:
To reach levels of reliability comparable to those required by high safety standards, it is imperative to supply circuits and electronic computing systems with appropriate mechanisms to reduce their vulnerability to radiation-induced soft errors. In this tutorial, we will discuss the key challenges, benefits, and impediments of assessing and mitigating the soft error reliability of circuits and systems, taking into account different levels of abstraction and approaches, varying from the use of ML to identify a particular source of error (e.g., software or hardware component), circuits techniques, to real laser-induced and neutron radiation experiments. To illustrate the relevance of each soft error assessment and mitigation approach, case studies covering safety-critical systems and resource-constraint IoT devices, applications, libraries/APIs and deep neural network characteristics will be discussed. This tutorial will also present circuitlevelmitigation techniques and a framework that supports fast and early soft error assessment, diagnosis, and susceptibility reduction evaluation by applying different mitigation techniques.
Bios
Luciano Ost is currently a Faculty Member with Loughborough University’sWolfson School - UK. He received his PhD degree in Computer Science from PUCRS, Brazil, in 2010. During his PhD, Dr Ost worked as an invited researcher at the Microelectronic Systems Institute of the Technische Universitaet Darmstadt (from 2007 to 2008) and at the University of York (October 2009). After completing his doctorate, heworked as a research assistant (2 years) and then as an assistant professor (2 years) at the University ofMontpellier II in France. He has authored a book and more than 90 papers. His research is devoted to advancing hardware and software architectures to improve life-critical and multiprocessing embedded systems’ performance, security, and reliability. Dr Ost delivered a tutorial at the IEEE ICECS conference in 2021 as well as invited talks and keynotes on various research topics not only at Universities (e.g., Berkeley 2018, University of York 2021) but also at Industrial (e.g., Arm Research Summit 2018), research conferences (e.g., International Embedded Systems Symposium 2019, SBESC 2020) andWebinars (e.g., IEEE CASS Talk 2021).
Ricardo Reis received a Bachelor degree in EE from Federal University of Rio Grande do Sul (UFRGS), Porto Alegre, Brazil (1978), and a Ph.D. degree in Microelectronics fromtheNational Polytechnic Institute of Grenoble (INPG), France (1983). Doctor Honoris Causa by the University of Montpellier (2016). He is a full professor at the Federal University of Rio Grande do Sul. His main research includes physical design automation, design methodologies, and fault tolerant systems. He has more than 700 publications including books, journals and conference proceedings. He was VP of IFIP (International Federation for Information Processing) and he was also president of the Brazilian Computer Society (2 terms) and vice-president of the Brazilian Microelectronics Society. He received the 2015 IEEE CASSMeritorious Service Award. He was vice-president of CASS for 2 terms (2008/2011). He is the founder of the Rio Grande do Sul CASS Chapter, which got the World CASS Chapter of The Year Award 2011, 2012, and 2018, and R9 Chapter of The Year 2013, 2014, 2016, 2017 and 2020. He is a founder of several events like SBCCI and LASCAS (CASS R9 Flagship Conference). He was General or Program Chair of several conferences like IEEE ISVLSI,
SBCCI, IFIP VLSI-SoC, ICECS, PATMOS. Ricardo was the Chair of the IFIP/IEEE VLSI-SoC SC, vicechair of the IFIP WG10.5 and he was Chair of IFIP TC10. He is founder of EMicro, an annually microelectronics school in Brazil. In 2002 he received the Researcher of the Year Award in the state of Rio Grande do Sul. He is a founding member of the SBC (Brazilian Computer Society) and also founding member of SBMicro (Brazilian Microelectronics Society). He was member of CASS DLP Program (2014/2015), and he has done more than 80 invited talks in conferences. Member of IEEE CASS BoG and IEEE CEDA BoG. Also member of the IEEE IoT Initiative. IFIP Fellow.
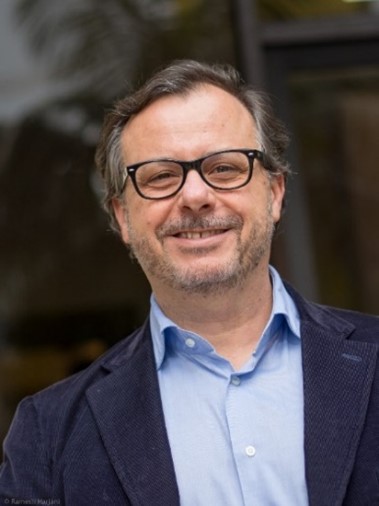
Alessandro Piovaccari
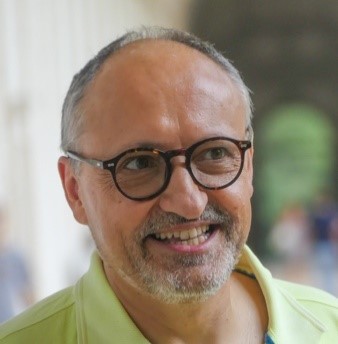
Marco Tartagni
Tutorial 3.1 A Simple and Intuitive Approach to Sensing, Sampling and Quantization
Abstract
This educational session is composed on two parts. In the first part, we will cover the topic of universal sensor design introducing a method for the design of any sensor acquisition chain is anyhow related to a few constraints (time, information, and power consumption). The method will show how framing the design along a few variables can enhance the overall performance and reduce the design time. We will also show how to optimize the segmentation and quantization of the sensing acquisition chain to maximize the resolution and the time response of the overall system.
In the second part, we will dive deeper and try to build an intuitive view of sampling and one-bit quantization on the specific topic of signal-noise interaction. Furthermore, we will see how this more intuitive and simpler view can help addressing problems that for an exact solution would require high-order math (like special functions) and provide approximate solutions which are useful in many applications, like determine the quantizer gain in single-bit delta-sigma converter or dynamic-element matching.
Bios
Alessandro Piovaccari is Visiting Fellow at UniBO, board member of the GSA CTO Council, the Center for Leadership Education at the Johns-Hopkins University, advisor for the Berkeley Skydeck and Silicon Catalyst startup incubators and newly appointed to VP of Conferences for the IEEE SSCS. He has more than 25 years of technical and management experience in the semiconductor industry, including CTO of Silicon Labs. He co-architected and led many wireless and IoT SoCs with a 4 billion devices shipments, including, the first single-chip TV tuner, used by nine of the top-ten TV makers and 70% market share. He holds PhD and Laurea degrees in EECS from UniBO and a Post-Grad (summa cum laude) in EECS from JHU. He is a Senior Member of IEEE and Lifetime Fellow at Silicon Labs, received an IEEE SSCS award in leadership, and holds 32 US patents, including a Patent of the Year award.
Marco Tartagni received the M.S. in EE in 1988 and the Ph.D. in EECS in 1993 from the University of Bologna, Italy. He joined the EE Department of Caltech, Pasadena, CA in 1992 as visiting student and in 1994 as research fellow, working on various aspects of analog VLSI for image processing. Since 1995 he is with the EE Department University of Bologna where he is currently Full Professor. From 1996 to 2001 he has been team leader in the joint lab between STMicroelectronics and University of Bologna working on intelligent CMOS sensors such as CMOS cameras and the world-first prototype of silicon based fingerprint capacitive sensor. He is co-recipient of the IEEE Van Vessem Outstanding Paper Award received at the 2004 ISSCC Conference. He has been European coordinator of the FP6 Receptronics project in Nanoelectronics area and he has been serving in the scientific committee of the IEEE Custom Integrated Circuit Conference (CICC) from 2017 to 2021.
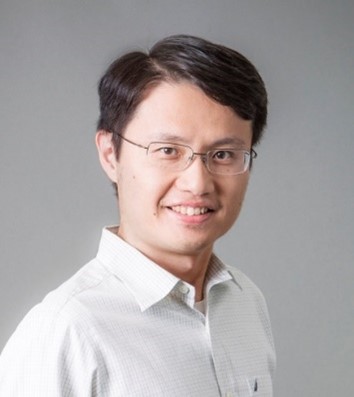
Mike Shuo-Wei Chen
Tutorial 3.2 Non-uniform Sampling ADCs
Abstract
Uniform sampling has been widely adopted in today’s circuit designs, ranging from data converters (ADC and DAC), and discrete-time signal processing (such as switched-capacitor filters). It is a well-developed processing technique that leads to many circuit architectures. However, Nyquist theory does not limit us to processing the samples at a uniform time grid so long as the average sample rate is sufficiently high, i.e., no loss of signal information. We refer data converters that sample at irregular time instants as non-uniform sampling (NUS) ADCs. In this tutorial, we will overview the basics of non-uniform sampling and the design tradeoffs for such ADC architecture. We will also examine several possible variations of NUS ADCs, and experimental results of silicon prototypes. Thanks to the unique properties of NUS, there are interesting possibilities for future circuit- and system-level architectural innovations.
BIO
Mike Shuo-Wei Chen is a professor in Electrical Engineering Department at University of Southern California (USC). He received the B.S. degree from National Taiwan University, Taipei, Taiwan, in 1998 and the M.S. and Ph.D. degrees from University of California, Berkeley, in 2002 and 2006, all in electrical engineering. As a graduate student, he proposed and demonstrated the asynchronous SAR ADC architecture, which has been adopted in industry today for low-power high-speed analog-to-digital conversion products. After joining USC in 2011, he leads an analog mixed-signal circuit group, focusing on high-speed low-power data converters, frequency synthesizers, RF/mm-wave transceiver designs, analog circuit design automation, bio-inspired computing, non-uniformly sampled circuits and systems. From 2006 to 2010, he worked on mixed-signal and RF circuits for various wireless communication products at Atheros Communications (now Qualcomm). Dr. Chen was the recipient of Qualcomm Faculty Award in 2019, NSF Faculty Early Career Development (CAREER) Award, DARPA Young Faculty Award (YFA) both in 2014, Analog Devices Outstanding Student Award for recognition in IC design in 2006 and UC Regents’ Fellowship at Berkeley in 2000. He also achieved an honorable mention in the Asian Pacific Mathematics Olympiad, 1994. Dr. Chen has been serving as SSCS Distinguished Lecturer, an associate editor of the IEEE Solid-State Circuits Letters (SSC-L), IEEE Transactions on Circuits and Systems II: Express Briefs (TCAS-II), as well as a TPC member in IEEE Solid-State Circuits Society conferences, notably the IEEE International Solid-State Circuits Conference (ISSCC), IEEE Symposium on VLSI Circuits (VLSIC), IEEE European Solid-state Devices and Circuits Conference (ESSCIRC) and IEEE Custom Integrated Circuits Conference (CICC).
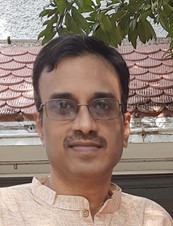
Shanthi Pavan
Tutorial 3.3 Precision Continuous-Time Delta-Sigma Data Converters
Abstract
Precision delta-sigma converters (i.e those that achieve 16+ bits resolutions over more than 10x the audio bandwidth) have typically been realized using discrete-time circuitry. This talk motivates the use of continuous-time delta-sigma modulation in such applications. Power-efficient, high-resolution continuous-time delta-sigma modulators need to consider several effects (some second-order, and others not-so-second-order) that might seem harmless in converters that attempt a more modest in-band noise spectral density. Examples of such effects include flicker noise, layout-routing resistance and DAC inter-symbol-interference. This talk aims to give insights into these effects and describe techniques that address the formidable challenge of designing such converters. A practical design example will be used to place things in perspective. It will also demonstrate that these techniques can be readily applied to higher speed designs.
Bio
Shanthi Pavan obtained the Sc.D degree from Columbia University, New York in 1999. After working in the industry for few years, he returned to his alma mater Indian Institute of Technology-Madras, where he is now the NT Alexander Institute-Chair Professor of Electrical Engineering. Dr.Pavan is the recipient of several awards, and is the author of Understanding Delta-Sigma Data Converters (second edition), with Richard Schreier and Gabor Temes), which received the Wiley-IEEE Press Professional Book Award for the year 2020. Dr.Pavan has served as the Editor-in-Chief of the IEEE Transactions on Circuits and Systems: Part I - Regular Papers, and on the editorial boards of both parts of the IEEE Transactions on Circuits and Systems. He has served on the technical program committee of the International Solid State Circuits Conference, and been a Distinguished Lecturer of the Solid-State Circuits and Circuits-and-Systems Societies. He currently serves as the Vice President of Publications for the IEEE Solid-State Circuits Society, and on the editorial boards of the IEEE Journal of Solid-State Circuits and the IEEE Solid-State Circuits Letters. He is a fellow of the Indian National Academy of Engineering, and an IEEE fellow.
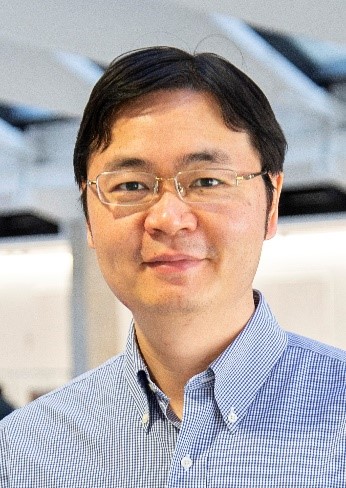
Nan Sun
Tutorial 3.4 Noise-Shaping SAR ADCs
Abstract
SAR is widely used for medium resolution applications due to its simplicity, scaling compatibility, and low-power consumption. However, its power efficiency degrades as the resolution increases due to its tight requirement on the comparator noise and the exponentially growing capacitor DAC array. By contrast, DS ADC is a popular architecture for high-resolution applications. Taking advantage of noise shaping, it can achieve high resolution with a low-resolution quantizer and DAC. However, it typically requires the use of op-amps that are power hungry and scaling unfriendly. This talk will present latest noise-shaping (NS) SAR ADCs that aim to combine the merits of SAR and DS ADCs while simultaneously obviating their drawbacks. It includes both cascade of integrators with feed-forward (CIFF) and error-feedback (EF) NS-SAR architectures. It covers various types of hardware implementations, including both active and passive loop filters and adders. It also discusses interesting extensions of NS-SAR ADCs, including time-interleaved NS-SAR and continuous-time (CT) DS ADC with NS-SAR quantizer.
Bio
Nan Sun is Professor with Tsinghua University since 2020. He was Assistant and then tenured Associate Professor with University of Texas at Austin. He received B.S. degree from Tsinghua University in 2006, and Ph.D. degree from Harvard University in 2010. Dr. Sun received the NSF Career Award in 2013, and the IEEE SSCS New Frontier Award in 2020. He has published 30+ JSSC papers and 50+ ISSCC/VLSI/CICC/ESSCIRC papers. He has graduated 24 PhD students, 10 of whom are professors at top universities in the US and China. He serves as TPC co-chair for CICC and TPC member for ASSCC. He was Associate Editor of TCAS-I, and a Guest Editor of JSSC. He also serves as Distinguished Lecturer for both IEEE Circuits-and-Systems Society and IEEE Solid-State Circuits Society.
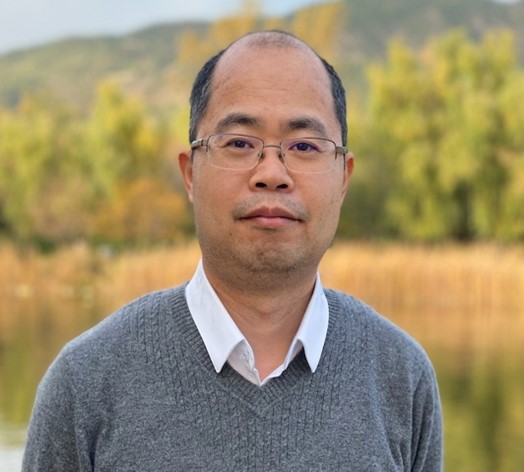
Zhibo Chen
Tutorial 4.1 Advances in Cross-Domain Image Compression
Abstract
In this tutorial, we will introduce the recent progress in generative image compression, which targets on learning a compression model effeciently storing images with low bitrates while producing perceptually high-fieldlity reconstructions. It has drawn increasing attention and made tremendous development in recent years because of better perceptual quality than traditional image compression algorithms at equal bitrates. We therefore conduct this tutorial of the analysis, methodology, and related applications of generative image compression to clarify the main progress the community has made. Specifically, we first briefly introduce the two most representative generative models, variational autoencoders (VAEs) and generative adversarial networks (GANs), applied for generative image compression. Second, we explore the developing area of generative image compression, including existing methods and potiential directions. In summary, our tutorial will cover latest works and the progress in community, which will help the audiences with different backgrounds better understand the recent progresses in this emerging research area.
Bio
Dr. Zhibo Chen received the B. Sc., and Ph.D. degree from Department of Electrical Engineering Tsinghua University in 1998 and 2003, respectively. He is now a full professor in University of Science and Technology of China. His research interests include image and video compression, visual quality of experience assessment, learning based visual signal representation and coding. He has more than 160 publications and over 80 granted patent applications. Some of his standard proposals have been adopted in MPEG/VCEG on video coding and ITU-T P.1202 on video quality assessment. He won the prestigious National Natural Science Award (second class) in 2018. He is IEEE senior member, Secretary of IEEE Visual Signal Processing and Communications Technical Committee (VSPC-TC). He has served as Associate Editor of IEEE Trans. on Circuits and Systems for Video Technology, Guest Editor for the IEEE Open Journal of Circuits and Systems, TPC chair of IEEE PCS 2019 and organization committee member of ICIP 2017 and ICME 2013, served as TPC member in IEEE ISCAS and IEEE VCIP.
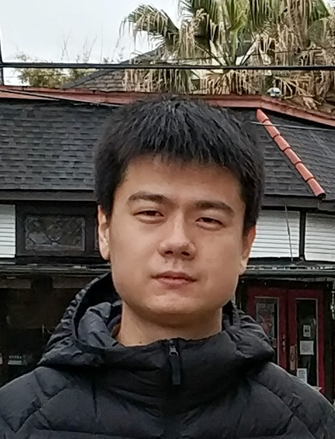
Jianxin Lin
Tutorial 4.2 Advances in Cross-Domain Image Generation
Abstract
Over the past several years, we have witnessed the impressive progress of cross-domain image generation based on deep learning approaches. It is attracting increasing attention because of its promising performance on a wide range of computer vision and image processing applications. In this tutorial, we will introduce a comprehensive overview of cross-domain image generation. Specifically, we categorize the cross-domain image generation problem into two main sets of tasks, i.e., supervised cross-domain image generation tasks and unsupervised/self-supervised cross-domain image generation tasks. We then provide a detailed taxonomy of the cross-domain image generation based on the different ways of designing model architecture, model optimization and sources of information, such as few-shot image generation or multi-modal image generation.
Bio
Jianxin Lin received the B.E. and Ph.D. degrees from University of Science and Technology of China (USTC) in 2015 and 2020. He is currently an associate professor at the School of Computer Science and Electronic Engineering, Hunan University, Changsha, China. He has published over 20 papers on related conferences and journals. His research interests include image and video processing, synthesis and understanding.
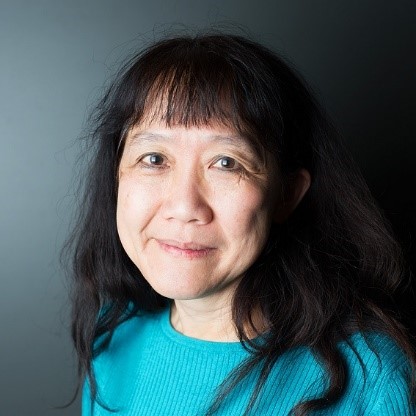
Shih-Chii Liu
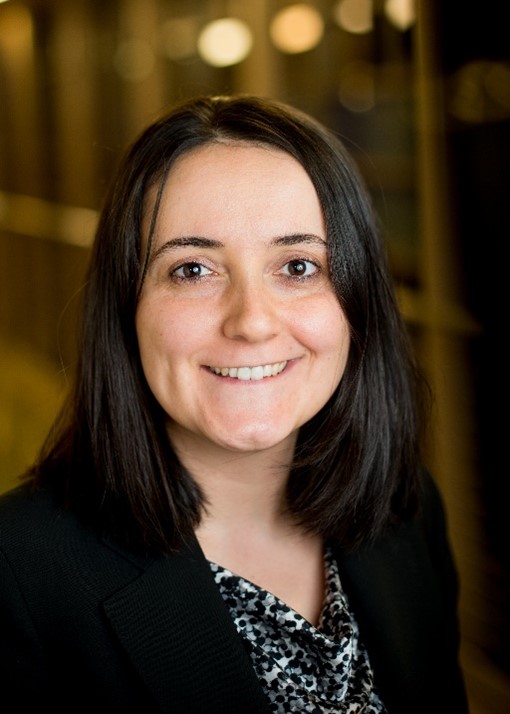
Duygu Kuzum
Tutorial 4.3 Neuromorphic Spiking Sensors, Network Accelerators, Non-volatile Memory Devices for Low-Power Edge Applications - Part I
Abstract
Neuromorphic spiking sensors including the event-driven Dynamic Audio Sensor (DAS) and Dynamic Vision Sensor (DVS) event camera are inspired by the functionality of the biological retina and cochlea equivalents. The asynchronous outputs of these event-driven sensors can enable always-on sensing at possible low-latency system-level response time than conventional sampled sensors for Internet of Things (IoT) and Brain-Machine Interface (BMI) applications. Recent developments in deep networks, spiking networks, analog and in-memory computing and non-volatile novel memory devices have led to very low-power neuromorphic systems that combine these sensors and networks for these application domains. Through supervised and unsupervised learning methods from deep learning and neuroscience fields, we show configurations of these systems and equivalent hardware to achieve high accuracy and low latency on benchmark tasks. The novel memory devices help to reduce power from off-chip memory access and can additionally, support different learning algorithms useful for adaptive neuromorphic systems. Analog In-memory computing methods reduce energy required to implement matrix multiplies, nonlinearities and other signal-processing operations over digital systems
Bios
Shih-Chii Liu is professor at the Faculty of Science, University of Zurich. She co-leads the “Sensors” group (www.sensors.ini.uzh.ch) at the Institute of Neuroinformatics, University of Zurich and ETH Zurich. Her research focus is on the design of low-power neuromorphic asynchronous spiking auditory and vision sensors, bio-inspired computing circuits, event-driven deep neural network processors and their use in neuromorphic artificial intelligent systems. Dr. Liu is past Chair of the IEEE CAS Sensory Systems and Neural Systems and Applications Technical Committees. She is current Chair of the IEEE Swiss CAS/ED Society and was general co-chair of the 2020 IEEE Artificial Intelligence for Circuits and Systems conference. She received the 2020 Misha Mahowald Award for Neuromorphic Engineering and is co-author of two textbooks on neuromorphic systems: Analog VLSI: Circuits and Principles (MIT Press) and Event-Based Neuromorphic Systems (Wiley).
Duygu Kuzum received her Ph.D in Electrical Engineering from Stanford University in 2010. She is currently an Associate Professor in the Electrical and Computer Engineering Department at University of California, San Diego. Her research focuses on development of nanoelectronic synaptic devices for energy-efficient neuro-inspired computing. Her group applies innovations in nanoelectronics to develop new technologies, which will help to better understand circuit-level computation in the brain. She is the author or coauthor of over 50 journal and conference papers. She was a recipient of a number of awards, including Texas Instruments Fellowship and Intel Foundation Fellowship, Penn Neuroscience Pilot Innovative Research Award (2014), Innovators under 35 (TR35) by MIT Technology Review (2014), ONR Young Investigator Award (2016), IEEE Nanotechnology Council Young Investigator Award (2017), NSF Career Award (2018), NIH NIBIB Trailblazer Award (2018), and NIH New Innovator Award (2020).
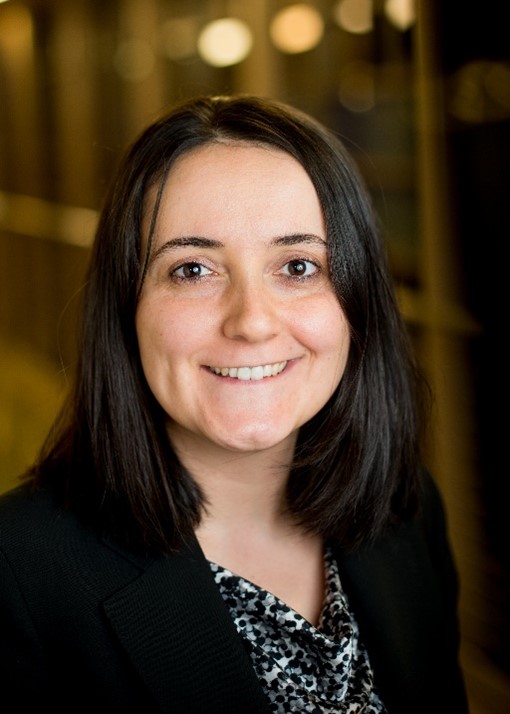
Duygu Kuzum
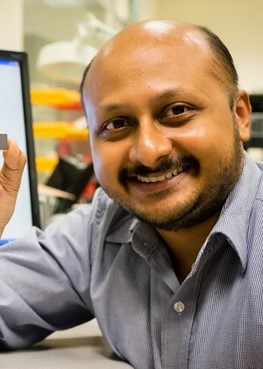
Arindam Basu
Tutorial 4.4 : Neuromorphic Spiking Sensors, Network Accelerators, Non-volatile Memory Devices for Low-Power Edge Applications - Part II
Abstract
A hallmark of brain-inspired computing is the intricately intermixed memory and compute architecture that strongly contrasts with conventional von Neumann ones with memory separated from computing elements. Digital processing element array architectures partially resolve this issue. An even more radical shift is offered by the In-memory computing (IMC) architectures where processing is performed entirely within the memory array with only the results being readout.
This tutorial will discuss two broad types of IMC based on Non-volatile and volatile memories. Analog computing techniques for accelerating matrix-vector multiplications using non-volatile RRAM will be the focus in the first half of the tutorial. The second half will deal with SRAM based in-memory image processing for dynamic vision sensors (DVS) event cameras and discuss particular case studies of traffic monitoring for the internet of video things.
Bios
Duygu Kuzum received her Ph.D in Electrical Engineering from Stanford University in 2010. She is currently an Associate Professor in the Electrical and Computer Engineering Department at University of California, San Diego. Her research focuses on development of nanoelectronic synaptic devices for energy-efficient neuro-inspired computing. Her group applies innovations in nanoelectronics to develop new technologies, which will help to better understand circuit-level computation in the brain. She is the author or coauthor of over 50 journal and conference papers. She was a recipient of a number of awards, including Texas Instruments Fellowship and Intel Foundation Fellowship, Penn Neuroscience Pilot Innovative Research Award (2014), Innovators under 35 (TR35) by MIT Technology Review (2014), ONR Young Investigator Award (2016), IEEE Nanotechnology Council Young Investigator Award (2017), NSF Career Award (2018), NIH NIBIB Trailblazer Award (2018), and NIH New Innovator Award (2020).
Arindam Basu received the B.Tech. and the M.Tech. degrees in electronics and electrical communication engineering from IIT Kharagpur in 2005,and the MS degree in mathematics and the Ph.D. degree in electrical engineering from the Georgia Institute of Technology, Atlanta, in 2009 and 2010, respectively. He is a Professor at City University of Hong Kong and was a tenured Associate Professor at NTU, Singapore earlier. His research interests include bioinspired neuromorphic circuits, non-linear dynamics in neural systems, low-power analog IC design, and programmable circuits and devices. Dr. Basu received the Prime Minister of India Gold Medal in 2005 from IIT Kharagpur. He was a Distinguished Lecturer of the IEEE Circuits and Systems Society for the 2016–2017 term. He received several awards including the MIT Technology Review’s TR35 Asia Pacific in 2012, the 40 under 40 award from Georgia Tech Alumni Association in 2021 and several best papers in IEEE conferences. He serves as an Associate Editor in a number of journals and is currently the Chair of IEEE CASS Technical Committee on Neural Systems and Applications.
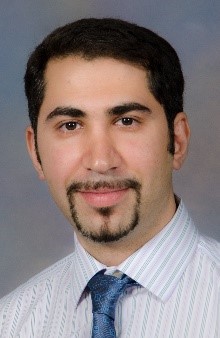
Nima Maghari
Tutorial 5.1 IC Security and Assurance Module: Mixed-Signal Approaches to IC Security
Abstract
Electronic counterfeiting is a longstanding problem with nontrivial impacts in many sectors. The sale of counterfeits results in substantial economic losses to the electronics industry, reportedly as high as$169B annually. To address these issues, in the last decade and so a large amount of research has been done to secure hardware. Security features such as physically unclonable function (PUF), hardware metering, and obfuscation have been developed to protect hardware from threats. Detection and avoidance techniques for IC counterfeiting and hardware Trojan have been introduced to protect the IC supply chain. Till now, most of the research has focused on digital techniques to tackle these challenges. In this talk, I will present several analog/mixed-signal approaches to IC security, including PUFs and camouflaged gates for IP protection which at their core, leverage analog mixed-signal techniques.
In this module, we will cover (1) IP protection using Physically Unclonable Functions (PUFs); (2) Performance merits of PUFs (3) design challenges and noise vs entropy (4) passive and active entropy sources (5) approaches to leverage new sources of entropy such as passive layers in silicon including metals and Vias, and active elements such as gate leakage (6) using positive feedback for Bimodal distribution to improve the bit error rate and stability; (7) Digital IP protection using camouflage gates (8) overhead and cost associated with the IP protection; (9) challenges to camouflage digital IPs at system level.
Bio
Nima Maghari received the B.S. degree in electrical engineering from the University of Tehran, Iran, in 2004 and the Ph.D. degree in electrical engineering from Oregon State University in 2010. He is currently an associate professor at the department of electrical and computer engineering, University of Florida, Gainesville. From 2004 to 2006, he was with IC-LAB, University of Tehran, where he was involved with audio delta-sigma converters and low-voltage bandgap references. In 2008 he was recipient of CICC-AMD outstanding student paper award. He has served as an Associated Editor of IEEE Transactions on Circuits and Systems-I, IET Electronics Letters and the technical program committee of IEEE CICC as Data Converter Sub-Committee Chair. He is on the editorial board of Journal of Solid-State Circuit Letters. He has published more than 70 conference and journals papers in IEEE and IEE. His research interests include high performance analog-to-digital converters, delta-sigma modulators, synthesizable analog circuits, low-power low-voltage regulators, and analog security and counterfeit detection.
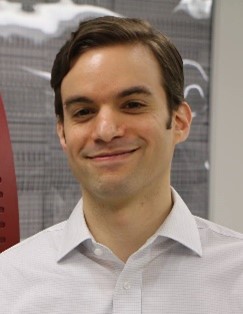
Domenic Forte
Tutorial 5.2 Counterfeit IC Detection and Avoidance
Abstract
Counterfeit electronic components are electronic parts that are misrepresented in terms of their authenticity, class, and/or quality. Due to their substandard specifications and quality, they represent a significant danger if incorporated into safety- and mission-critical systems such as military/defense, space and aircraft navigation, life support, etc. Counterfeit electronics can also infringe the legitimate producer's trademark rights, impact the market reputation of semiconductor and electronics companies, harm the world economy, and finance criminals. Reports of counterfeits have grown over the last several decades because of critical infrastructure’s reliance on commercial-off-the-shelf (COTS) components and obsolete components as well as the rate at which electronics are being discarded. Unfortunately, this problem has shown no signs of slowing down. In fact, experts have warned that counterfeit electronics will be exacerbated by the reduction in precious metal imports and the pandemic-induced chip shortage.
In this module, we will cover (1) Counterfeit electronic part sources, motivation, and risks; (2) Counterfeit IC type taxonomy (recycled, remarked, overproduced, cloned, etc.); (3) Counterfeit defect taxonomy (procedural, mechanical, environmental, and electrical); (4) Counterfeit detection taxonomy (physical, electrical, design-for-anti-counterfeit; (5) Basic parametric/functional tests for counterfeit component detection; (6) More advanced detection approaches that explicitly target counterfeit SoCs, memories (SRAM, DRAM, and Flash), field programmable gate arrays (FPGAs), and AMS ICs; (7) Design-for-anti-counterfeit primitives such as physical unclonable functions (PUFs) and silicon odometers, and their benefits; (8) International standards and commercially available tools; (9) Barriers to the adoption of counterfeit detection tests and avoidance circuits; and (10) Future directions in research, infrastructure, standards, and policy-making to improve the accuracy, cost, scalability, and adoption of counterfeit mitigation techniques.
Bio
Domenic Forte is an Associate Professor and the Steven A. Yatauro Faculty Fellow with the Electrical and Computer Engineering Department at University of Florida, where he also serves at the Director of the FICS Research SeCurity and AssuraNce (SCAN) lab. His research covers the domain of hardware security from nano devices to printed circuit boards (PCBs) where he has nearly 200 publications. Dr. Forte is a senior member of the IEEE, a member of the ACM, and serves on the organizing committees of top conferences in hardware security such as HOST and AsianHOST. He also serves and has served on the technical program committees of DAC, ICCAD, NDSS, ITC, ISTFA, BTAS, and many more. Dr. Forte is a recipient of the Presidential Early Career Award for Scientists and Engineers (PECASE), the NSF Faculty Early Career Development Program (CAREER) Award, and the ARO Young Investigator Award. His research has also been recognized with best paper awards and nominations from IJCB, ISTFA, HOST, DAC, and AHS.
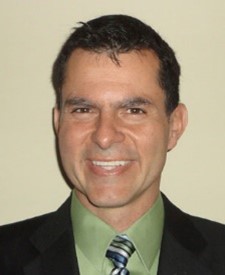
Gabriel A Rincon-Mora
Tutorial 5.3 How to Stabilize and Control DC–DC Switched-Inductor Power Supplies, Part 1
Tutorial 5.4 How to Stabilize and Control DC–DC Switched-Inductor Power Supplies, Part 2
Abstract
Switched-inductor dc–dc power supplies are pervasive in consumer electronics: in cellular phones, tablets, laptops, chargers, cars, and so on. This is because these voltage regulators supply loads at steady voltages without losing much of the power they draw. Unfortunately, controlling them is a challenge. They close analog feedback loops that switch an inductor into a load with digital signals. Engineers connect a capacitor across this load to supply the dynamic power the inductor cannot provide. This capacitor is small in modern consumer applications, so it cannot supply much power without drooping the output too much. So in addition to managing the mixed-signal complexities of a switched loop, the system needs to respond quickly (with high bandwidth) and reliably (stable feedback operation) to variations in load and the input that supplies the system. This tutorial explains how voltage- and current-mode loops control and stabilize these switching dc–dc converters. With this understanding in mind, the material shows how pulse-width-modulated (PWM), hysteretic, and constant-time peak/valley loops switch the inductor, offset the output, and respond to load and input variations. While some of these concepts can be found in literature, they are largely abstract and algebraic, incomplete, and usually spread over several sources. This presentation, on the other hand, is concise, complete, and full of design insight.
Bio
Gabriel A. Rincón-Mora is Fellow of the National Academy of Inventors, Fellow of the IEEE, and Fellow of the Institution of Engineering and Technology. He's been a Professor at Georgia Tech since 2001, was a Visiting Professor at National Cheng Kung University in 2011–2019, Adjunct Professor at Georgia Tech in 1999–2001, and Design Team Leader at Texas Instruments in 1994–2003. He was inducted into Georgia Tech's Council of Outstanding Young Engineering Alumni and named one of "The 100 Most Influential Hispanics" by Hispanic Business magazine. Other distinctions include the National Hispanic in Technology Award, Charles E. Perry Visionary Award, Three-Year Patent Award, Orgullo Hispano Award, Hispanic Heritage Award, State of California Commendation Certificate, IEEE Service Award, and included in "List of Notable Venezuelan Americans" in Science. His body of work includes 11 books, 8 handbooks, 4 book chapters, 42 patents issued, over 190 articles, 25 educational videos, over 26 commercial power-chip products released to production, and over 150 keynotes/speeches/seminars.